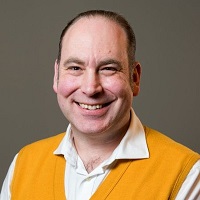
In this special guest feature, Stephen Amstutz, Head of Strategy and Innovation, Xalient, discusses the role of AI in the shift from network monitoring to observability, highlighting the benefits of AI observability in limiting downtime, protecting brand reputation, and ultimately saving money! Stephen is a results-driven, hardworking professional, capable of understanding complex matters outside his direct area of expertise. He enjoys the challenge of finding the right technical solution to meet a customer’s business challenges. Stephen’s background in electronics provided him with a strong analytical foundation which he’s maintained through 20+ years’ experience in design, implementation and support of various IT infrastructures.
In today’s world, the volume of data and network bandwidth requirements are growing relentlessly. So much is happening in real-time as businesses adapt and advance to become more digital, which means the state of the network is constantly evolving. Meanwhile, users have high expectations around applications – quick loading times, look and feel visually advanced, with feature-rich content, video streaming, and multimedia capabilities – all of these devour network bandwidth. With millions of users accessing applications and mobile apps from multiple devices, most companies today generate seemingly unmanageable volumes of data and traffic on their networks.
Networks are dealing with unmanageable volumes of data
In this always-on environment, networks are completely overloaded, but organizations still need to deliver peak performance from their network to users with no degradation in service. But traffic volumes are growing, and this is bursting networks at peak hours, akin to the L.A. 405; no matter how many lanes are added to the freeway, there will always be congestion problems during the busiest periods.
As an example, we’re seeing increasing need for rail operator networks to handle video footage from body-worn cameras, in order to cut down on anti-social behavior on trains and at stations. However, this directly impacts the network, with daily uploads of hundreds of video files consuming bandwidth at a phenomenal rate, yet the operators still need to go about their day-to-day operations while countless hours of video footage are uploaded and processed.
This is a good example of where AI and ML can and is helping organizations take a proactive stance on capacity and analyze whether networks have breached certain thresholds. These technologies enable organizations to ‘learn’ seasonality and understand when there will be peak times, implementing dynamic thresholds based on the time of day, day of the week, etc., as a result. AI helps to spot abnormal activity on the network, but now this traditional use of AI/ML is starting to advance from ‘monitoring’ to ‘observability’.
So, what is the difference between the two?
Monitoring is more linear in approach. Monitoring informs organizations when thresholds or capacities are being hit, enabling organizations to determine whether networks need upgrading. Whereas observability is more about the correlation of multiple aspects and context gathering and behavioral analysis.
For example, where an organization might monitor 20 different aspects of an application for it to run more efficiently and effectively; observability will take those 20 different signals and analyze the data making diagnostics with various scenarios presented. It will leverage the rich network telemetry and generate contextualised visualizations, automatically initiating predefined playbooks to minimize user disruptions and ensure quick restoration of service. This means the engineer isn’t waiting for a call from a customer reporting that an application is running slow. Likewise, the engineer doesn’t need to log in and run a host of tests, and painstakingly wade through hundreds of reports, but instead can quickly triage the problem. It also means network engineers can proactively explore different dimensions of these anomalies rather than get bogged down in mundane, repetitive tasks.
This delivers clear benefits to the business by reducing the time teams spend manually sifting through and analyzing realms of data and alerts. It leads to faster debugging, more uptime, better performing services, more time for innovation, and ultimately happier network engineers, end-users and customers. Observability correlation of multiple activities enables applications to operate more efficiently and identify when a site’s operations are sub-optimal with this context delivered to the right engineer at the right time. This means a high volume of alerts is transformed into a small volume of actionable insights.
Machines over humans
Automating this process, and using a machine rather than a human, is far more accurate because machines don’t care how many datasets they must correlate. Machines build hierarchies, and when something in that hierarchy impacts something else, the machine spots certain behaviors and finds these faults. The more datasets that are added, the more of a picture this starts to build for engineers who can then determine whether any further action is required.
Let’s touch on another real-life example. We are currently in discussions with a large management company who own and manage gas station forecourts. They have 40,000 gas stations, and each forecourt has roughly 10 pumps, equating to 400,000 gas pumps across the US. Their current pain point is a lack of visibility into the gas pumps and EV chargers connected to the network. As a result, when a pump or charger is not working, they might only become aware of this following a customer complaint, which is far from ideal.
The network telemetry that we are gathering, and that behavior analysis, means we are developing business insights, not just network insights. We can see if a gas pump stops creating traffic, which triggers a maintenance request to go and fix the pump. This isn’t a network problem, but the network traffic can be leveraged to look for the business problem. This is a use case for gas pumps and EV chargers but imagine how many other network-connected devices there are in factories or production facilities worldwide that could be used in a similar way.
Getting actionable insight quickly
This is where our AIOps solution, Martina, predicts and remediates network faults and security breaches before they occur. Additionally, it helps to automate repetitive and mundane tasks while proactively taking a problem to an organization in a contextualised and meaningful way instead of simply batting it across to the customer to solve. Martina discovers issues with recommendations around tackling the problem, ensuring that organizations always have high-performing resilient networks. In essence, it essentially makes the network invisible to users by providing customers with secure, reliable, and performant connectivity that works. It provides a single view of multiple data sources and easily configurable reporting so organizations can get insights quickly.
Executives and boards want their network teams to be proactive. They won’t tolerate poor network performance and want any service degradation, however slight, to be swiftly resolved. This means that teams must act on anomalies, not thresholds, to understand behavior to predict and act ahead of time. They need fast MTTD and MTTR because poor-performing networks and downtime impact brand reputation and ultimately cost money! This is where proactive AI/ML observability really comes into its own.
Sign up for the free insideBIGDATA newsletter.
Join us on Twitter: https://twitter.com/InsideBigData1
Join us on LinkedIn: https://www.linkedin.com/company/insidebigdata/
Join us on Facebook: https://www.facebook.com/insideBIGDATANOW
"network" - Google News
December 03, 2022 at 10:00PM
https://ift.tt/caXV8ek
How AI Enables Organizations to Move from Network Monitoring to Proactive Observability - insideBIGDATA
"network" - Google News
https://ift.tt/k0UVgzN
Shoes Man Tutorial
Pos News Update
Meme Update
Korean Entertainment News
Japan News Update
No comments:
Post a Comment